What is Data Analytics?
Data analytics refers to the process of examining and interpreting raw data to extract meaningful insights, patterns, and trends. It involves using various techniques and tools to analyze data in order to make informed decisions, support business strategies, and discover actionable information. Data analytics is utilized across various fields, including business, science, healthcare, finance, marketing, and more, to turn data into valuable knowledge.
There are different types of data analytics:
- Descriptive Analytics: This involves summarizing historical data to provide an overview of what has happened in the past. It focuses on understanding the current state of affairs and does not involve predictions or insights into the future.
- Diagnostic Analytics: Diagnostic analytics aims to identify the reasons behind certain outcomes or events. It involves analyzing data to understand why a particular situation occurred, often by digging deeper into patterns and correlations.
- Predictive Analytics: Predictive analytics uses historical data to make predictions about future events or outcomes. It involves applying statistical algorithms and machine learning techniques to forecast trends, possibilities, and probabilities.
- Prescriptive Analytics: This type of analytics goes beyond predicting future outcomes and suggests actions to achieve specific goals. It combines data analysis with optimization techniques to recommend the best course of action.
Data analytics typically involves several stages:
- Data Collection: Gathering relevant data from various sources, which can include databases, spreadsheets, APIs, sensors, and more.
- Data Cleaning and Preparation: Raw data often contains inconsistencies, errors, and missing values. This stage involves cleaning and transforming the data into a usable format.
- Exploratory Data Analysis (EDA): Exploring the data to identify patterns, relationships, and initial insights. Visualization tools are often used in this stage to help understand the data better.
- Data Modeling: This involves applying statistical or machine learning models to the data to identify patterns, correlations, and trends. These models can range from simple regression analysis to complex machine learning algorithms.
- Interpretation and Insights: Analyzing the results of the data modeling stage to draw meaningful insights and conclusions. This is where the actual value of data analytics is realized.
- Visualization and Reporting: Presenting the findings in a visually appealing and understandable manner. Data visualizations, charts, graphs, and reports help communicate the insights to stakeholders effectively.
Data analytics is a crucial component of data-driven decision-making, allowing organizations and individuals to make informed choices based on evidence rather than intuition alone. It plays a pivotal role in enhancing operational efficiency, identifying opportunities for growth, and solving complex problems across various domains.
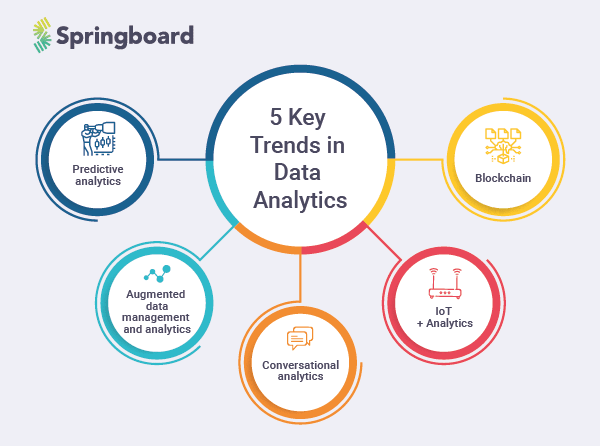
Data analytics is applied in a wide range of industries and fields due to its ability to extract insights, patterns, and trends from large datasets. Here are some areas where data analytics is commonly applied:
- Business and Marketing:
- Market Analysis: Analyzing customer preferences, market trends, and competitive landscapes to make informed business decisions.
- Customer Segmentation: Dividing customers into distinct groups based on their behaviors, demographics, and preferences to tailor marketing strategies.
- Sales Forecasting: Predicting future sales based on historical data, market conditions, and other relevant factors.
- Churn Prediction: Identifying customers at risk of leaving a service or product, allowing for targeted retention efforts.
- Finance and Banking:
- Risk Assessment: Evaluating credit risk and fraud detection by analyzing transaction data and customer behaviors.
- Portfolio Management: Optimizing investment strategies by analyzing market data, historical performance, and economic indicators.
- Fraud Detection: Identifying unusual patterns or anomalies in financial transactions to detect and prevent fraudulent activities.
- Healthcare and Medicine:
- Clinical Research: Analyzing patient data to identify correlations between treatments and outcomes, aiding in drug development and clinical trials.
- Disease Prediction: Using patient data to predict disease risks and outcomes, enabling early interventions and personalized treatments.
- Healthcare Operations: Optimizing hospital operations by analyzing patient flow, resource utilization, and staff performance.
- Manufacturing and Supply Chain:
- Supply Chain Optimization: Analyzing supply chain data to improve inventory management, demand forecasting, and production efficiency.
- Quality Control: Monitoring and analyzing production data to ensure product quality and identify defects.
- Predictive Maintenance: Using sensor data to predict equipment failures and maintenance needs, minimizing downtime.
- E-commerce and Retail:
- Recommendation Systems: Suggesting products to customers based on their browsing and purchase history, improving the user experience.
- Inventory Management: Analyzing sales data to optimize inventory levels and prevent stockouts or overstocking.
- Price Optimization: Setting optimal pricing strategies by analyzing market dynamics, competitor pricing, and customer behavior.
- Energy and Utilities:
- Smart Grid Management: Analyzing energy consumption patterns to optimize distribution, reduce waste, and improve overall efficiency.
- Renewable Energy: Using data to predict energy production from renewable sources, optimizing their integration into the grid.
- Transportation and Logistics:
- Route Optimization: Analyzing traffic and logistical data to find the most efficient routes for deliveries and transportation.
- Fleet Management: Monitoring vehicle performance and maintenance needs through sensor data analysis.
- Demand Prediction: Predicting transportation demand to allocate resources effectively and improve service availability.
These are just a few examples of the many areas where data analytics is applied. As technology advances and more data becomes available, the potential for data analytics to provide insights and drive decision-making continues to grow.